Cheat sheet: A comprehensive guide to AI terminology
Artificial intelligence as a discipline consists of hundreds of individual technologies, concepts, and applications. These terms have become increasingly important as STEM education expands and there is a boom in practical household and consumer-facing applications for the technology.
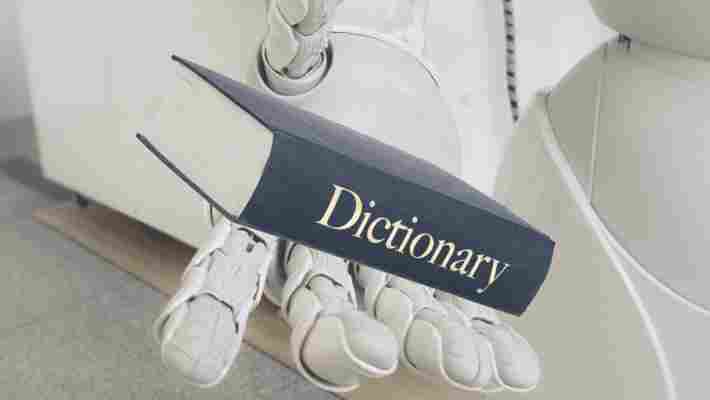
Despite that, there is a lack of consistency in how many AI concepts are discussed, not just at the STEM education level, but in popular entertainment, science writing, and even at times in scientific journals. To address this, we need to standardize how we describe AI and its many subsets, and accurately define these terms both in general and specific to individual technologies and applications of those technologies. We discuss some of the most commonly misused and what they really mean.
Through decades of alternating optimism and disappointment in the prospects of artificial intelligence , AI experts like Nick Bostrom, Demis Hassabis, Geoffrey Hinton, and Ray Kurzweil have pushed through challenges in funding and declining public interest to define what we know of the science today. From the basics of abductive reasoning to the utilization of deep learning algorithms , the language used to describe AI has developed over the course of six decades to represent a complex combination of concepts and ideas.
All that time has created a problem, though, with so many terms now commonly misused in STEM education, popular entertainment, and even in scientific journals where certain parts of the vernacular have become interchangeable with one another. From conflation of common terms like machine learning and AI to the use of more advanced concepts like behavioral analysis, it’s likely that you are currently using some of these common phrases incorrectly.
Let’s take a close look at what they really mean.
Artificial intelligence
Artificial intelligence (AI) is a blanket term that often refers to a suite of technologies and concepts. When we talk about self-learning machines in any form, it’s usually tagged with “AI.” What AI really refers to is “weak AI” or the simulation of human intelligence to complete a very narrow task . Broader ability to perform tasks within the realm of human intelligence is artificial general intelligence (AGI) and is more commonly what we are referring to when describing chatbots, voice systems, or medical bots.
Synthetic intelligence
This is often used interchangeably with artificial intelligence but is nuanced in that it emphasizes that machine intelligence can be a distinct form of intelligence, separate from our own. It is therefore not artificial, but genuine in its own way.
Machine learning
The AI boom of the last eight years often refers to breakthroughs here, in the ability of a machine to process large volumes of data to develop or improve upon a specific skill. Machine learning is the process by which artificial systems learn to beat humans at Go , analyze large volumes of call center data, or personalize voice assistant responses.
Deep learning
Deep learning is often used in conjunction with machine learning to describe the process by which machines take in large data sets to “learn” new things. Specifically, deep learning refers to the use of an artificial neural network (ANN) to simulate the structure and process of the human brain. It is machine learning that utilizes layered algorithms to identify and learn from specific patterns in big data.
Supervised learning
Supervised learning is used in instances where the system needs to develop a map that can accurately predict the outcome based on the input. To do this, input and the desired output are provided to the system. This form of learning is most often used in retrieval-based AI, as opposed to unsupervised learning in which the system is only provided input data without any corresponding output to measure against.
There are some advantages to be gained from the supervised model, but also several limitations. Because the input and output expectations are limited (and often defined by humans) such systems don’t always react well to unexpected input.
Speech recognition
Speech recognition has been around in several forms for decades and consists of various technologies that can recognize and transpose human language into text on a computer. There are several subsets of speech recognition, including automation speech recognition (ASR), speech to text (STT), and more modern variants that incorporate into voice recognition systems.
Affective computing
Affective computing refers to the subset of disciplines through which computers can not only recognize but the process and analyze human emotions. It is often referred to also as emotion AI or artificial emotional intelligence and sits at the crosssection of psychology and computer science. It is what enables us to utilize behavioral recognition technologies in voice algorithms.
Automated reasoning
Automated reasoning is both a subset of artificial intelligence and a cross-section of philosophy, theory, and practical computer science. It is the study of the ways in which computer programs can reason through problems automatically, without input from a human user. It often incorporates different technologies and processes to accomplish these goals and is most often used to describe a concept than a specific technology.
Machine vision
Machine vision (MV) is the combination of hardware and software technologies to enable a computer system to analyze image-based data often in the industrial space. It allows for automatic inspections, process controls, and is one of the underlying technologies of self-guiding robots. There is no one technology that makes up MV—it is a combination of integrated systems to solve individual problems in the real world. As a practical application, it is most often used in industrial automation, security, and self-driving vehicles .
Behavioral analysis
Behavioral analysis in the traditional sense is a natural science by which scientists study the factors that directly influence behavior, including biological, experiential, environmental, and even pharmaceutical. It’s often linked to fields of psychology and was until very recently a very difficult science due to the sheer volume of data points present in human behavior.
AI-driven behavioral analysis takes a similar approach but uses the full array of signals: the content of one’s speech, the movement of their body, the timbre of their voice. Everything is collected and analyzed to identify key behavioral patterns.
Emotionally intelligent data
Deep learning is being used to map the array of human emotions and supplement big data with emotionally intelligent data. It is a way of exploring not only the content of interaction but the emotional state of those having it.
This is most pronounced in areas of high human interaction, such as customer service or sales. While phone calls have always been recorded for screening and agent evaluation, AI-systems can now capture millions of emotion-based data points that can help better understand why someone reacts the way they do on a call. From precursors to anger to confusion or outside factors that can influence the call, this is a great way to better understand and respond to someone’s needs, even before they voice them.
Behavioral intent prediction
Companies spend billions of dollars every year to better understand customers and attempt to predict their intentions. Behavioral intent prediction dates back to 1980 when Paul Warshaw published a new model for evaluating and predicting consumer actions. The number of variables such a model requires to have actionable accuracy, however, has only recently become feasible.
Today’s AI systems utilize emotionally intelligent data to parse human speech and better understand what drives decisions. It evaluates how humans engage with voice assistants and other AI systems every day. Alexa, for example, now uses Hunches , a skill that can identify patterns in user activity based on thousands of unique factors.
Emotion recognition
AI’s ability to recognize and respond to emotion has evolved as ANNs and the deep learning tools that utilize them have advanced. Drawing from tens of thousands of unique human characteristics in speech, systems can identify dozens of unique emotions with high levels of accuracy.
While facial recognition is easier to fool—humans have been hiding their emotions on their faces for millennia—the voice is a much more accurate gauge. Cadence, volume, word choice, the length of individual pauses—all of these can be used to recognize and measure the intensity of individual emotions in speech.
Voice technology
Voice technology refers to the growing suite of tools that leverage speech recognition to provide a new interface for human use of machines. The most common are personal voice assistants: Alexa, Siri, or Cortana. But voice technology is being leveraged in other unique ways. Integration of emotion recognition and behavioral intent prediction makes voice technology a viable tool in customer service and sales applications for real-time feedback on calls.
It provides passive feedback on patient status in hospitals. It can be customized to fit the needs of professionals – allowing doctors to reduce the time spent on administrative tasks for example. Voice technology is more than just the voice on your phone—it is a new form of user interface that is becoming an increasingly important part of our digital lives.
Understanding the future of AI and voice technologies
AI will continue to grow and adapt to unique use cases across dozens of industries and personal technologies. It’s being integrated into the general population’s everyday activities and becoming a more persistent component of how we interact with the world. As it does, the language we use to describe it needs to be more precise. There may not be a need to properly represent AI-specific terminology today, but the more integrated it becomes with our lives, the more vital it becomes for us to nail down the specific language we use to describe it (even if we’re not working in STEM).
This article was originally published by Rana Gujral on TechTalks , a publication that examines trends in technology, how they affect the way we live and do business, and the problems they solve. But we also discuss the evil side of technology, the darker implications of new tech and what we need to look out for. You can read the original article here .
Leave a Comment